- Rios-Torres, J., and Malikopoulos, A.A., “An Overview of Driver Feedback Systems for Efficiency and Safety,” Proceedings of 2016 IEEE 19th International Conference on Intelligent Transportation Systems, pp. 667-674, 2016 (pdf).
- Malikopoulos, A.A. and Aguilar, J.P., “An Optimization Framework for Driver Feedback Systems,” IEEE Trans. Intell. Transp. Syst., 14, 2, pp.955-964, 2013 (pdf).
- Malikopoulos, A.A. and Aguilar, J.P., “Optimization of Driving Styles for Fuel Economy Improvement,” Proceedings of 2012 15th International IEEE Conference on Intelligent Transportation Systems, 194-199, 2012 (pdf).
Driver Information Systems
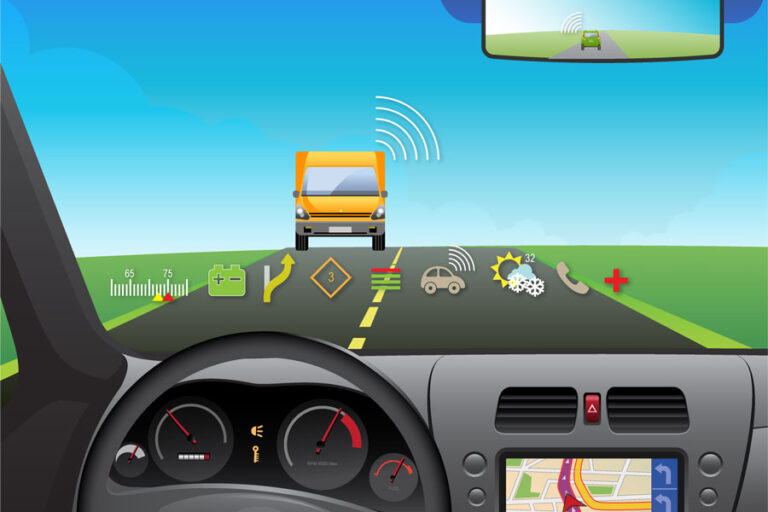
Driver feedback systems have the potential to improve driving safety and efficiency by providing instructions to drivers aimed at improving their driving style. There is already a rich body of available literature devoted to the derivation of energy efficient speed profiles to develop driver feedback or eco-driving systems. While most of them can be applied to any type of vehicle, their effectiveness will be maximized if their formulation involves the dynamics of the particular vehicle powertrain configuration. The variation in fuel consumption for different driving styles is significant, so developing a means of improving driver behavior to maximize fuel economy is another way to reduce fuel consumption in existing fleets. In previous research, we have investigated driving style factors that have a major impact on fuel economy. More specifically, we’ve developed an optimization framework with a real-time feedback system, including visual instructions to enable drivers to alter their driving styles in response to actual driving conditions to improve fuel efficiency. Realizing a more eco-driving style can contribute significantly to sustainable mobility.
As the necessity for connected and automated vehicles (CAVs) is becoming pervasive, it seems that driver feedback systems could be potentially combined with CAVs and provide solutions for improving both safety and efficiency for the entire transportation network. It is apparent that new approaches are needed that can take advantage of external information collected in real time, to maximize the benefits of eco-driving systems. The advent of CAVs provides the opportunity for such new approaches as they foster the development of improved systems to monitor traffic conditions and the design and implementation of optimal strategies as a result of the available global data and information. However, many challenges have still to be addressed before having a massive deployment of fully automated vehicles. It is expected, that CAVs will penetrate in the market slowly and interact with non-autonomous vehicles.
Two critical questions need to be addressed: 1) how to take advantage of the connected environment and driver assistant systems to provide instructions to drivers? and 2) how to account for the uncertainty produced by drivers who do not follow the instructions to guarantee the safety of the traffic network? Investigating methods to habilitate autonomous and non-autonomous connected vehicles to safely interact under realistic scenarios is critical to fully exploit the opportunities to improve efficiency and reduce emissions, offered by intelligent transportation systems. Driver responses to external information related to real-time conditions, social and news media can have a significant impact in the traffic network efficiency and safety and thus, it is imperative that eco-driving systems involve some level of driver behavior prediction.
Given the previous extensive research into how best to link human controllers with other complex systems, it would seem prudent to consider how results from those studies might apply to the present context. We suggest that, given the current state of the art, there are many opportunities to improve the basic understanding of how real-time information, drivers-in-the-loop and the driver-vehicle interface can be optimally integrated. We also expect that the most impactful results will be integrated by studies that bridge across multiple traditional disciplines, such as mathematics, computational simulation, statistics, mechanical and electrical engineering, measurement science, neuroscience and human factors engineering.