Seminars by Andreas Malikopoulos
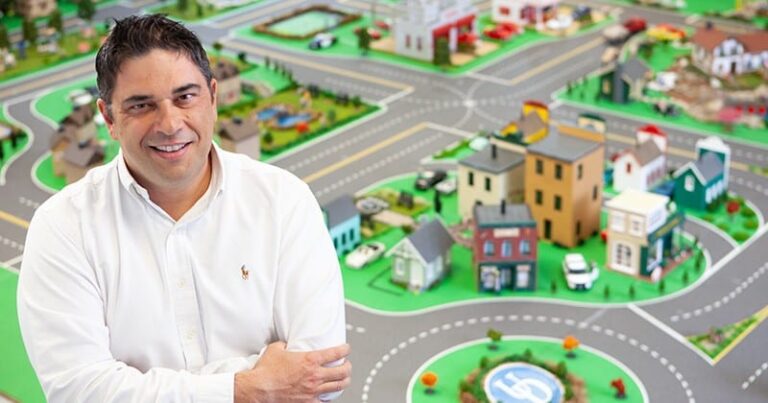
Dr. Andreas Malikopoulos delivered several talks entitled “Separation of Learning and Control for Cyber-Physical Systems” at Rutgers University on October 22, at Boston University on November 19, at Stanford University on December 3, and at MIT on December 8.
Cyber-physical systems (CPS), in most instances, represent systems of subsystems with an informationally decentralized structure. To derive optimal control strategies for such systems, we typically assume an ideal model, e.g., controlled transition kernel. Such model-based control approaches cannot effectively facilitate optimal solutions with performance guarantees due to the discrepancy between the model and the actual CPS. On the other hand, in most CPS there is a large volume of data with a dynamic nature which is added to the system gradually in real time and not altogether in advance. Thus, traditional supervised learning approaches cannot always facilitate robust solutions using data derived offline. By contrast, applying reinforcement learning approaches directly to the actual CPS might impose significant implications on safety and robust operation of the system. In these talks, Dr. Malikopoulos presented a theoretical framework founded at the intersection of control theory and learning that circumvents these challenges in deriving optimal strategies for CPS. In this framework, we aim at identifying a sufficient information state for the CPS that takes values in a time-invariant space, and use this information state to derive separated control strategies. Separated control strategies are related to the concept of separation between the estimation of the information state and control of the system. By establishing separated control strategies, we can derive offline the optimal control strategy of the system with respect to the information state, which might not be precisely known due to model uncertainties or complexity of the system, and then use learning methods to learn the information state online while data are added gradually to the system in real time. This approach could effectively facilitate optimal solutions with performance guarantees in a wide range of CPS applications such as emerging mobility systems, networked control systems, smart power grids, cooperative cyber-physical networks, cooperation of robots, and internet of things.